Which proportion of missing values in the data is allowed?
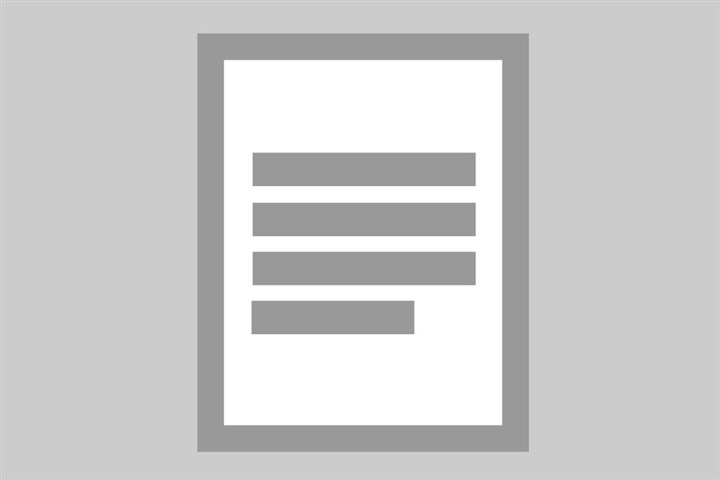
In the literature, it has been recommended not using data with more than 50% missing counts, or sometimes even not more than 20%. But a rule of thumb on the proportion of missing counts tolerated is hard to give, because this depends on the fit of the statistical model applied. The more missing counts are present in the data, the more one has to rely on the statistical model to estimate (impute) missing counts. If the model fits, the imputed values are expected to be close to the observed values, so the higher the proportion of missing values is allowed to be. TRIM allows the user to fit different models in order to find a proper model, with better indices and often smaller standard errors as a result. Anyway, do not remove those sites with many missing counts from the data as an attempt to improve the data!
In case of lack-of-fit, the quality of the imputations as well as the resulting indices remains questionable. Usually, however, a low quality of the indices due to lack-of-fit is already expressed in the standard errors of the indices. That is because TRIM converts any lack-of-fit into higher standard errors when overdispersion is taken into account.