Structural Time Series Models for Consumer Confidence indicators
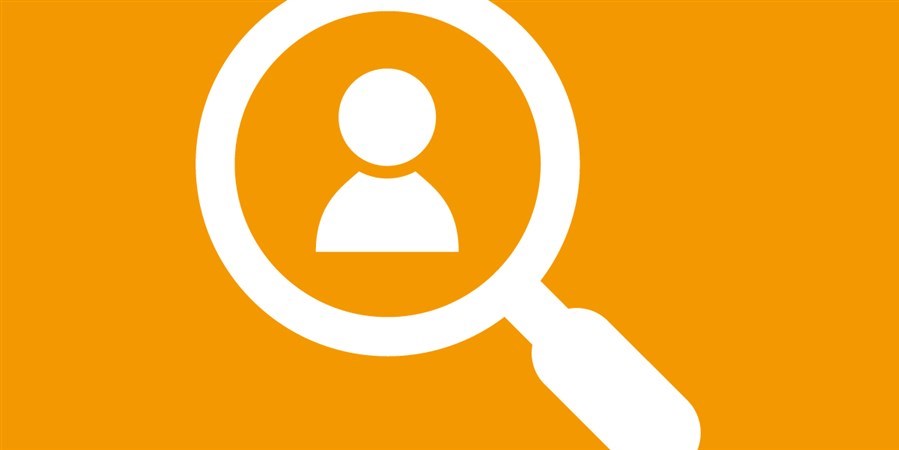
The structural time series model improves the accuracy of the direct estimates, since it provides a better separation of measurement errors and sampling errors from estimated target parameters.
The standard errors for the month-to-month changes are clearly smaller under the time series model.
A second problem addressed in this paper is related to the transition to a new survey process in 2017. Structural time series models in combination with a parallel run are applied to estimate discontinuities induced by the redesign. A backcasting algorithm is developed to construct uninterrupted input series for the structural time series model.
This inference method facilitated a smooth transition to a new survey design and resulted in uninterrupted published series about consumer confidence that date back to 1986.
The method is implemented for the production of official monthly figures on consumer confidence in the Netherlands.