Multivariate density estimation by neural networks
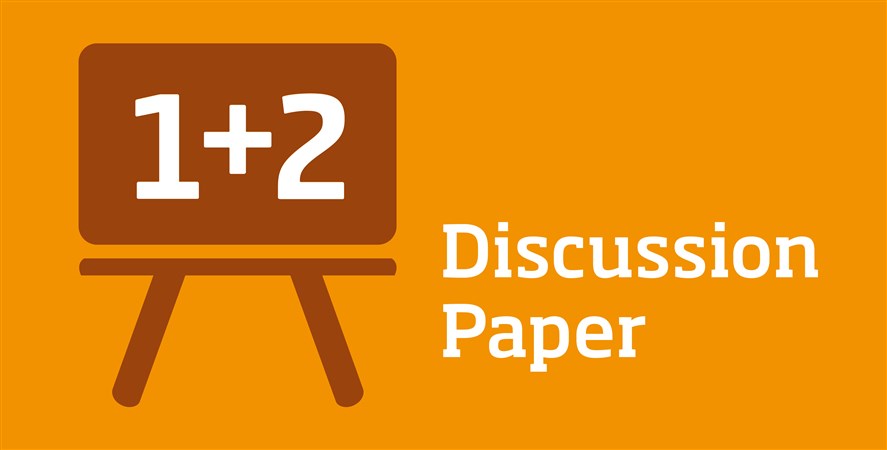
Erratum:
After publication of an earlier version of this paper, we received feedback that there were several incorrect references to related methods in the literature. These errors are corrected in the current version, and explained in the pdf at the bottom of this page. We are grateful to professor E. Trentin from University of Siena for his valuable feedback.Existing methods in the literature can be used to approximate continuous probability distributions only. The methodology in this paper can be used to estimate multivariate continuous and discrete probability distributions. Through simulations, it is shown how the methodology performs for a probability mixture of normal distributions, a probability mixture of generalized extreme value distributions, a probability mixture of Poisson distributions, bivariate Poisson distribution, bivariate normal distribution and a trivariate normal distribution.