Towards motives behind mobility
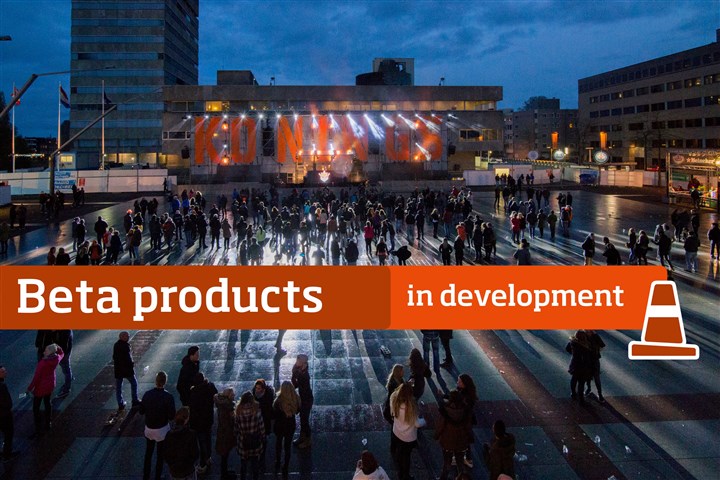
Statistics Netherlands (SN) is studying new data sources and approaches to provide deeper insights into people moving from A to B. Policymakers are faced with increasing complex decisions regarding accessibility of services, air pollution, new mobility solutions and costs. As a result, information to support good decision making is in high demand.
What is the plan?
We use a stepwise approach in which new data sources and register based data sources are combined with survey data. Examples of these new data sources are traffic loop data and anonymised data from mobile phone providers. Register based data sources are the register on wages (in Dutch: the ‘Polis’ registration), the education register and the register of addresses of utility services (malls, day-care centres).
In this approach we use the information in register data as a proxy for different travel motives: the Polis for commuting to work, the education register for travel to the place of study and the register of addresses of utility services (malls, day-care centres, cinemas) for a mixture of other motives.
Combining the addresses from the population register with destination points from the other registers results in origin-destination matrices, in which each cell represents the number of people who might travel between the origin and destination point for a given travel motive.
With travel motives derived from these register sources we combine these data with actual traffic counts from new data sources. This should provide a more detailed insight in reasons why people are travelling from A to B, the choices they make for departure times, and the mode of transport.
What has been done in this step?
For this beta product we use the dataset that is also used for our existing commuting statistic. In this statistic, for each combination of two city districts (one for place of residence and one for place of work) the number of commuters between those districts is counted and the distance between the two city-centres is derived. The distance is based on the shortest route by car from district-centre to district-centre. The dataset for this statistic is the result of combining data from the region-survey (into the number of employees for a company in a municipality) with data from the ‘Polis’ and the company register. An important step in this approach is that (anonimised) persons working for a specific company are attributed to the local establishment of a company. For this, a proximity algorithm is used. In this algorithm the baseline is the number of people working for a company within a municipality. With this algorithm we can approximate the working location of a person.
In this beta product we visualised these data in a new way. The visualisation shows the maximal contribution of commuters based on Polis data on each stretch of road. We again use the detailed dataset which we use to calculate routes (again the shortest route by car) between the centre of the origin city-district and the destination city-district. The result of this step was a collection of routes: one route for each origin-destination combination. We can use the collection of routes to calculate the contribution of commuting, under certain conditions, to traffic intensity for specific stretches of road.
Each route is in itself a collection of (shorter) stretches of road. Each stretch of road may occur in one or more routes. One person traveling from A to B, and another traveling from A through B to C both use the stretches of roads between A and B, so these stretches appear twice in the set. For each particular stretch of road, we counted the number of occurrences in the set of routes. We then multiplied this number by the number of commuters that are likely to take that route. The result is a projection of the traffic intensity per stretch of road you would expect from all people in the register moving from their home to their place of work (and vice versa).
The traffic intensity map
The visualisation shows the results of this first step, projected on the Limburg area. By clicking on the link below, an interactive map of the Limburg region opens. It shows some interesting patterns, that coincide with other congestion sources.
With this map we demonstrate the possibilities of register based data sources for mobility policy making. With this beta product we specifically want to establish the usefulness of this product for policy makers. For this reason, some basic assumptions were made that form the basis of the dataset we used and the map we made. In later steps, these assumptions need to be checked and, if possible, refined. The most important assumption is that we do not yet distinguish between modes of transport; for each working location we calculate a route that consists of public roads (as compared to rails, bustracks). In next steps we will work on distinguishing different transport modes, such as bike, car and public transport. Regarding the dataset: we do not know the exact working location of a person, we only know the company she or he works for and the companies' registered postal address (which can deviate for companies which have several sites). For the dataset the assumption is made that people travel to the nearest site in the city closest to the place of residence.
In this map, roads that are used by a larger number of commuters are differentiated from roads that are used by fewer commuters. On a higher zoom level (showing the entire Limburg area) deeper red colours indicate higher traffic intensity, lighter red colours lower traffic intensity. On a lower zoom level (showing for instance the Maastricht area) more roads are displayed, and the colour differentiation is substituted for thinner (lower traffic intensity) and thicker ( higher traffic intensity) lines.
Click on the picture to enter the “road use” map
We plan to extend this visualisation to a map of the Netherlands. In this new map we want to classify traffic intensity into different classes. That should help to reduce the number of road segments. We think an interesting feature in the map would be of ‘route filtering’. Such a filter should enable a user to filter for certain origins or destinations. Most probably those would be cities, but maybe some specific applications could be made with more lower level regional filtering options.
Next steps
Right now we are working on creating a similar map, based on public transport planners.
The dataset underlying the visualisation can be improved by comparing origin-destination data from registers with counts from new data sources coming from, for example, public transport, traffic loops and mobile phones. By confronting these datasets, assumptions on the choice of working location, choice of mode of transport, travel days and departure times can be tested. In this way, motives behind mobility can be attributed to a large part of measured travel data. Statistics Netherlands is looking for partners to optimally contribute knowledge and data on this topic.Safeguards to ensure privacy
The data about the working and living locations are aggregated by district. No information on individual routes is registered with this map.What do you think?
We would like to know your opinion on road use intensity maps and our ideas on feature development on it. This could be your general impression or specific points, either positive or negative.
We would also like to know what kind of use cases you have for these kinds of maps. Is a lower regional level useful? What do you think of filtering options?