Multilevel Hierarchical Bayesian vs. State Space Approach in Time Series Small Area Estimation: the Dutch Travel Survey
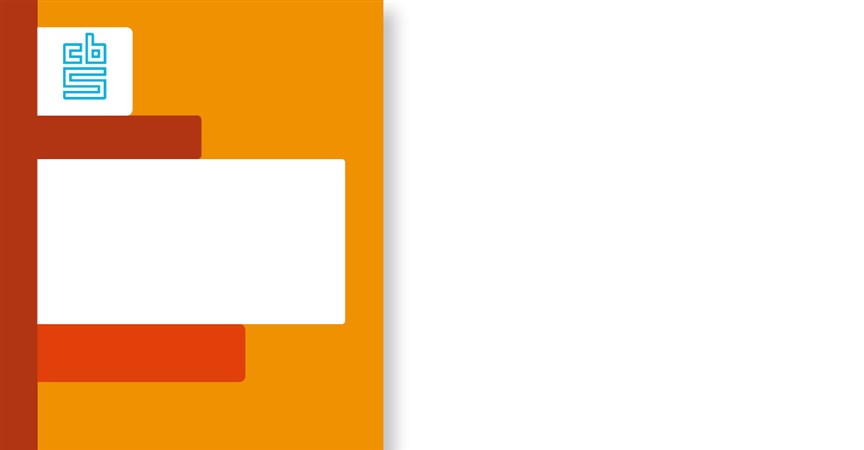
This study compares two different techniques in a time series small area application: state space models estimated with the Kalman filter with a frequentist approach to hyperparameter estimation, and multilevel time series models estimated within the hierarchical Bayesian framework. The application chosen is the Dutch Travel Survey featuring level breaks caused by the survey redesigns, as well as small sample sizes for the main publication domains. Both models require variances of the design-based domain estimates as prior information. In practice, however, only unstable estimates of the design-based variances are available. In this paper, excessive volatility and a possible bias in design-based variance estimates are removed with the help of a state space model. The multilevel and state space modelling approaches deliver similar results. Slight differences in model-based variance estimates appear mostly in small-scale domains and are due to neglecting uncertainty around the hyperparameter estimates in state space models, and to a lesser extent due to skewness in the posterior distributions of the parameters of interest. The results suggest that the reduction in design-based standard errors with the hierarchical Bayesian approach is over 50% at the provincial level, and over 30% at the national level, averaged over the domains and time.