Correcting for linkage errors in contingency tables
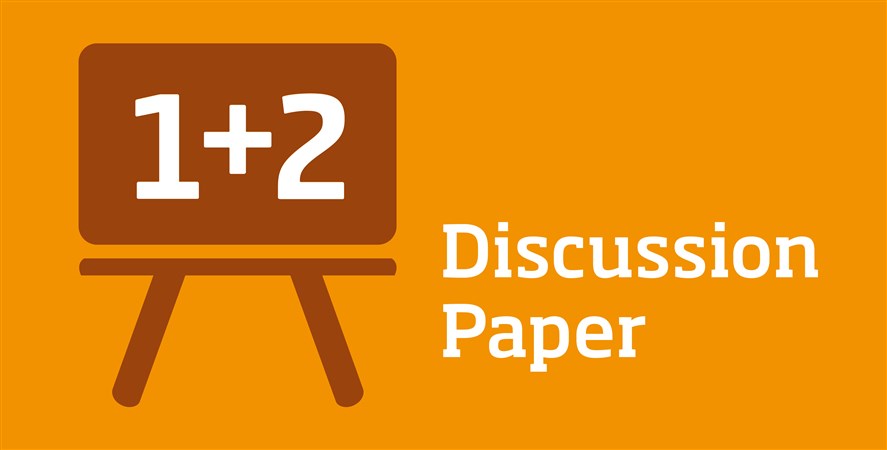
Record linkage aims to bring records together from two or more files that belong to the same statistical entity such as an individual or a business. In this paper, we focus on incorrectly linked pairs which result from records in two or more datasets being linked incorrectly due to errors, missing values or changes over time in the variables that are used in the matching procedure. It is well known that naïvely treating a probabilistically linked file as if there are no linkage errors leads to biased inference.
We present two approaches for compensating for linkage error when analysing a two-way contingency table for categorical data where one variable is coming from one file and the second variable is coming from the other file. One approach is an unbiased correction and we show that this often equates to the approach used by Chipperfield and Chambers (2015) under the exchangeable linkage error model, which is a widely used model for linkage errors and the one that we will adopt in this paper. The other approach is a biased correction, but which often leads to lower mean square error than the unbiased approach.
Under the exchangeable linkage error model, we will study the following fundamental questions: can a compensation approach for linkage error improve on the naïve approach, where linkage error is not compensated for, and, if so, under what conditions? To this end, we will compare the compensation approaches to the naïve approach. We will examine these three approaches in detail, both by means of an analytical study as well as by means of a simulation study. In particular, we examine estimation errors, bias, variance and mean square error of the three approaches. We show in this paper that the approach to take for a given situation depends on the characteristics of the table and whether the table shows dependent or independent attributes, and more specifically whether the particular cell of the table has a positive, negative or no association.